DeepSDF
type
status
date
slug
summary
tags
category
icon
password
Introduction
learned shape-conditioned classifier
- a continuous implicit surface
- a probabilistic auto-decoder
Modeling SDFs with Neural Networks
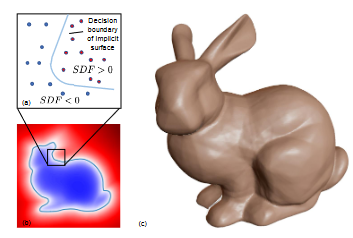
- 8 mlp
- 512-d
- ReLU and tanh
- weight-normalization instead of batch-normalization
- Weight Normalization是对网络权重W进行归一化(L2范数),使其方向与大小分离,然后用一个标量g和一个向量v来重写W。Batch Normalization是对网络某一层的输入数据进行归一化,使其均值为0,方差为1,然后用一个缩放因子γ和一个偏移因子β来重写归一化后的数据。
loss
𝕃(fθ(x), s) = |clamp(fθ(x), δ) − clamp(s, δ)|
clamp(x, δ) := min(δ, max( − δ, x))
Learning the Latent Space of Shapes
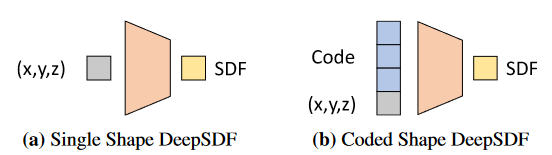
SDFs
auto-decoder
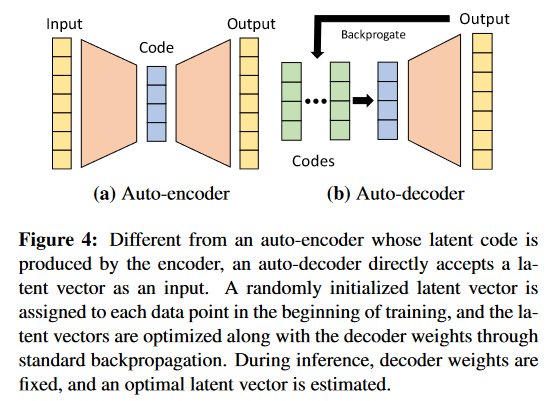
一个形状中的采样点
假设X符合联合分布
根据Maximum-a-Posterior最大后验估计
latent vector的概率
training
inference
fixing θ,训练新的latent vector
数据准备
对与每个3D 模型取一些列的采样点 (x, s),采样方式是个比较繁琐的过程 (假设是 watertight 的mesh 模型,先将模型 normalize到一个单位球,接着在表面采250000 个点,并保留其法向量,然后对250000 点加扰动,每个点扰动得到 2 个点,一共会有500000个扰动空间点,对这些空间点计算 SDF 值,计算方式就是从250000个表面点中找最近的点,然后计算符号与距离。